CERES
EO-based information for “smarter” agriculture and Carbon farming
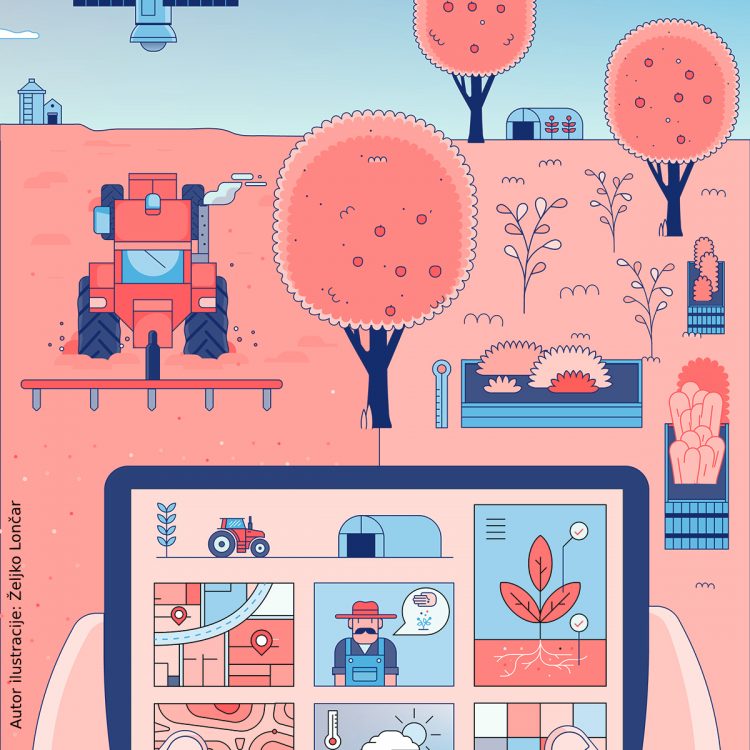
Project description
The CERES project deals with the development of tools based on Artificial Intelligence (AI) algorithms intended to support agricultural production and Carbon farming practices which result in carbon sequestration in soil and combat climate change. The idea of the project is to create models which would use big geospatial data from various sources – optical and radar satellite images (Copernicus missions), soil related data (in-situ, LandGIS, SoilGrids), accurate meteorological data and textual data available on internet portals intended for agriculture – to automatically generate new information which will help make timely and correct decisions in agriculture.
The models will therefore be developed for early identification of changes in crop growth, automated interpretation of the causes of the changes, yield estimation and estimation of Soil Organic Carbon, identification of tillage activities.
The use of AI in agriculture is a trend all over the world, however, in Serbia it should be especially important because agriculture is one of the crucial sectors of Serbian economy. This project will be an important step forward in the application of a wide range of relevant data generated on a daily basis and offering a huge potential for improving agricultural production and developing the concept of smart and regenerative agriculture.
OBJECTIVES
The goal of the CERES project is to develop a set of geospatial information products based on artificial intelligence algorithms and geoinformatics, together with remote sensing products, text mining, including soil sampling, designed to support information driven decisions in agriculture, increase profitability of the agricultural sector and support Carbon farming in Serbia.
To develop Identification of crop disturbance (disease, pests, drought, heil, storm)
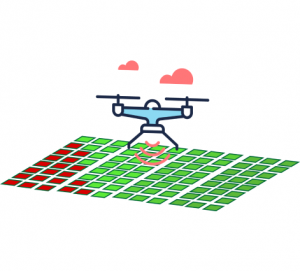
Earth Observation (EO) data will be utilised, processed and “translated” to valuable information related to the change on the agricultural parcel. The pattern recognition and early detection of crop disturbance will be based on the Artificial Neural Network (ANN). Training data, in situ data, will be collected in the context of CERES project, potential Copernicus in-situ data as well as from other European projects.
To develop crop yield estimation for main crop types (wheat, maize, barley, soya, sunflower)
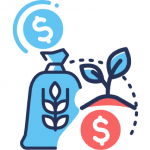
Crop yield estimation (prediction) is important for the analysis and comparison of field productivity but also it allows farmers to decide at farm level whether selling the product or leading it to the storage and for defining the logistics activities for enabling an effective transfer from farm to the industry. A Machine Learning (ML) crop specific models based on biophysical parameters, Vegetation Indices, radar and optical data and meteorological data will be developed.
To develop identification of tillage activities for regenerative agriculture
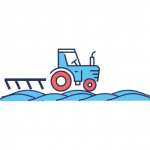
Carbon farming includes the cultivation techniques of regenerative agriculture that take carbon dioxide out of the atmosphere (where it causes global warming) and convert it into carbon-based compounds in the soil that aid plant growth. Within this objective, we will present an approach for mapping tillage activity changes by using Sentinel-1 and Sentinel-2 data, at high spatial resolution (100 m), by applying temporal change detection algorithm based on ANN based ML classifier.
To develop Soil Organic Carbon model for spatial interpolation
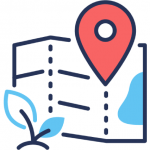
Soil Organic Carbon (SOC) is a major source of terrestrial carbon and also a key element for soil quality and fertility representing an important element of terrestrial ecosystems, due to its great potential to affect the climate, food security, and agricultural sustainability. The surface reflectance and vegetation indices derived from the optical satellite imaging (Sentinel-2), soil texture derived from radar imaging (Sentinel-1), climatic variables and terrain factors will be used as covariates in order to build a predictive model for SOC. Since SOC is a source of nutrients and is crucial for agricultural production, the estimated SOC stock should be very important in our models for agricultural management and yields estimates.
To develop methods for extracting information on agricultural events’ occurrence and yield estimates using the unstructured textual sources
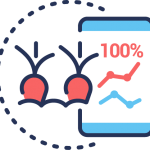
The information about undesirable agricultural events (calamity, drought, disease, pest attack, …) and yield estimates are often published in numerous local or state-wide news sites, agricultural reports, and social media profiles. Such information, if properly recognized, extracted and geolocated, could complement the available optical and radar EO data when building and validating different estimation models. The extracted information is especially valuable in cases when the primary data is noisy, corrupted or missing.
Therefore, it is necessary to apply different AI methods which include Natural Language Processing of Serbian language, Expert defined rules and ML techniques, to extract and classify the information about relevant, time-and-space determined events.
To generate high resolution daily temperature and precipitation maps

Temperature and precipitation data affect agricultural cropping decisions, cropping management decisions, irrigation scheduling, fire weather management, disaster preparedness, etc. High resolution daily temperature maps will be generated from freely available temperature observations using spatio temporal regression kriging. Spatio temporal interpolation will also be used to generate high resolution daily precipitation maps from freely available precipitation observations.